The new AI innovation equation for your business solutions
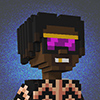
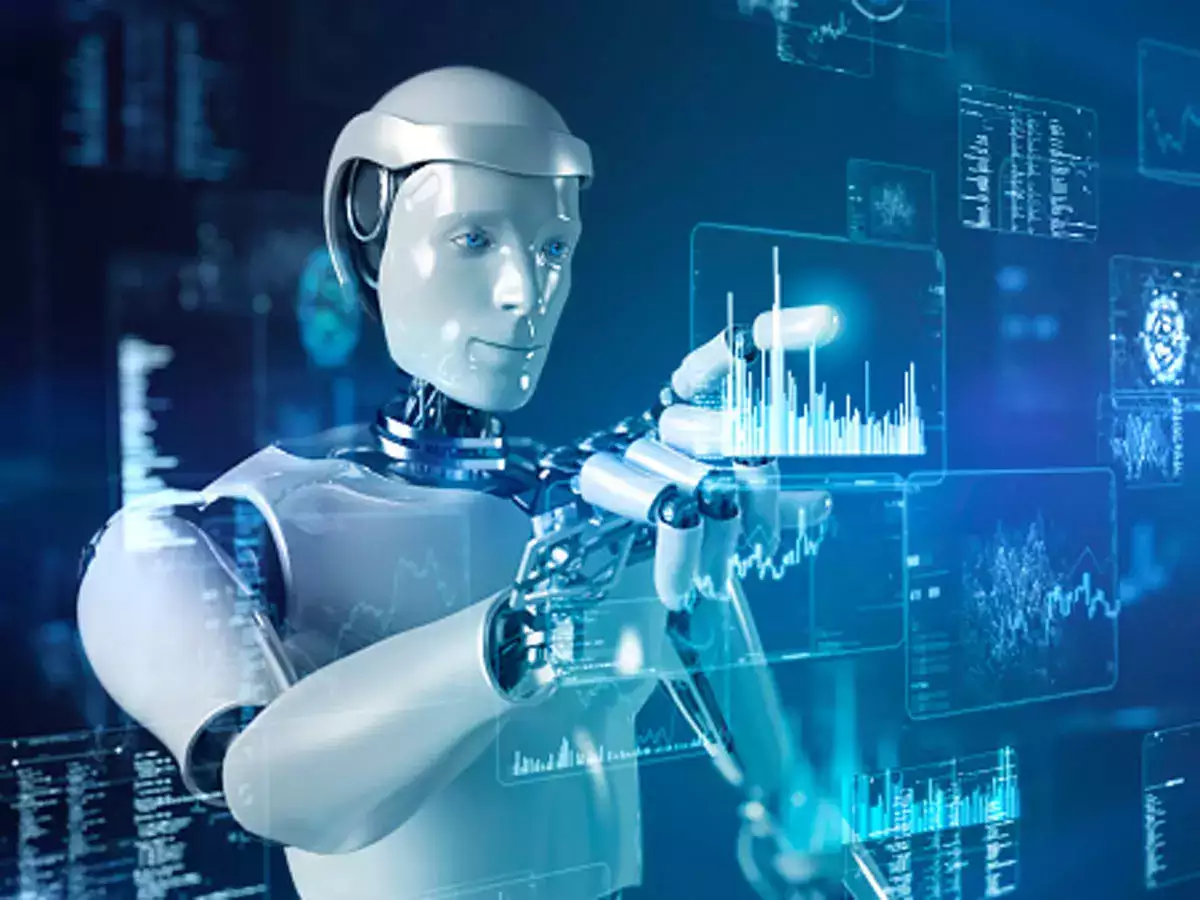
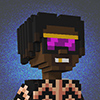
After decades of experiencing a slow burn, artificial intelligence innovation is now the hottest topic on the agendas of the world's leading technological companies.
What is fuelling this fire? Necessity. "Faced with a continual deluge of data, we required a new sort of system that learns and adapts, and AI now provides that," says Arvind Krishna, Senior Vice President of Hybrid Cloud and Director of IBM Research. "What was believed unthinkable a few years ago is rapidly becoming not only doable but also anticipated and required."
As a result, several tech giants, start-ups, and researchers have been racing to create artificial intelligence (AI) solutions that potentially give a competitive edge by boosting human intelligence.
Today's AI advancements would not be possible without the convergence of three factors that created the right equation for AI growth: the rise of big data, the emergence of powerful graphics processing units (GPUs) for complex computations, and the re-emergence of a decades-old AI computation model—deep learning.
Although this triumvirate has only scratched the surface of its potential, it is never too early to look ahead. We consulted 30 experts to examine some of the impetuses of the next generation of AI advancements. They found a new formula for the advancement of AI in the future, one in which existing variables receive a makeover and new variables inspire enormous jumps. Prepare for a formula that involves the emergence of little data, more efficient deep learning models, deep reasoning, new AI technology, and the advancement of unsupervised learning.
Rationale enters the equation.
Experts have little doubt that deep learning will continue to play a crucial role in the new AI growth equation, as it has in the past. "Deep learning is having a significant influence in a variety of fields, including voice, vision, and natural language processing. John Smith, manager of multimedia and vision at IBM Research, predicts that this trend will persist for a time.
However, deep learning has yet to demonstrate a strong capacity to assist computers with thinking, a talent they must acquire to improve the performance of many AI applications.
"Deep learning has been successful for well-defined problems with lots of labelled data, and it excels at perception and classification problems rather than real reasoning problems," says IBM Distinguished Researcher Murray Campbell, one of the architects of IBM's artificial intelligence chess master, DeepBlue. "The next great potential is to apply deep learning to reasoning as it has been used to perception and categorization."
IBM: Games, AI, and Cognitive Computing's Future
Watch the video (03:03)
The reasoning is necessary for a variety of cognitive activities, such as applying common sense, adapting to changing circumstances, simple planning, and making sophisticated judgments in a career. "Humans are aware that if an object is placed on a table, it will likely remain there unless the table is slanted. However, nobody writes it in a book; it's something implied. Aya Soffer, IBM's Director of AI and Cognitive Analytics Research, emphasizes that systems lack this common sense competence.
"If a legislature adopts a law in conformity, for instance, it is feasible that a newly elected legislature will repeal elements of that legislation. According to IBM Research's Chief Scientist for Compliance, Vijay Saraswat, you must embed into your system the core concept of human life that anything may change.
"Symbolic reasoning and alternate intelligence pathways will be required to develop more robust AI tools."
— Kevin Kelly, Wired co-founder and bestselling author of The Inevitable
Experts in artificial intelligence concur that we are still in the early stages of training systems to deeply think, with just a few examples of success in niche applications such as self-driving vehicles and a few professions. To attain a level of efficiency that permits extending reasoning capabilities across a larger range of applications, more effort remains.
"We have now reached the point where, after much work in tagging text, we can map natural language sentences to logical forms in certain domains. Saraswat of IBM explains that we may then utilize formalized reasoning processes to interact with these extracted formulae. "The primary issue is to significantly minimize the work required to get at these formulations in several domains."
Some AI experts are confident that the reasoning problem will be solved within the next five to ten years, and they note that deep learning may be part of the solution.
"The potential to create a vast thinking capacity is now within our reach. There are very strong indications that machine learning techniques can transform data into a form required for automated reasoning, according to Michael Witbrock, manager of cognitive research at IBM. "We have vast quantities of data, and while they may not be in the exact format you desire, there are strong indications that machine learning techniques can transform data into the format required for automated reasoning," Witbrock says that what is required is a method for massively scaling up reasoning calculations, similar to what GPUs did for neural networks.
Panel Discussion on Machine Learning Applications
Watch the video (43:18)
Deep learning gets a facelift
Deep learning is here to stay, but it will likely take on a new form in the next wave of AI advancements. Experts emphasize the necessity to train deep learning models more efficiently to apply them at scale to more complicated and diversified applications. This efficiency will be achieved in part through the use of "little data" and more unsupervised learning.
The introduction of tiny data
Deep learning models' neural networks must be exposed to massive volumes of data to learn a task. To train a neural network to detect an item, for instance, up to 15 million photos may be necessary. Acquiring datasets of this magnitude may be expensive and time-consuming, which slows down the training, testing, and refinement of AI systems.
And sometimes there is simply insufficient data available on a certain topic to feed a deep-learning model. "In the field of health, how many participants are there in clinical studies? Thousands, if you're fortunate, but even that will take years to acquire. According to IBM Cognitive Solutions Research Manager Costas Bekas, patients do not have the time to wait.
Researchers are optimistic that they will discover a feasible solution to the problem of training algorithms with less data. As a result, AI specialists anticipate a reversal of the "data" component in the AI development equation, with tiny datasets replacing large datasets as the primary drivers of new AI innovation.
Towards unsupervised learning
Current deep learning models need not just huge but also labelled datasets so that the machine understands what each data point represents. Humans are primarily responsible for labelling in supervised learning, a hard operation that slows down innovation increases costs, and may inject human bias into systems.
Even when labels are there, systems frequently require extra human guidance to learn. "A subject matter expert is providing the system with all of their knowledge. Michael Karasick, vice president of Cognitive Computing at IBM, explains that the process may be quite arduous for small and medium-sized enterprises.
Unsupervised learning, on the other hand, permits raw, unlabelled data to be utilized to train a system with minimal human intervention. "In unsupervised learning, the system interacts only with the environment. "It just observes the environment and learns from it," explains IBM's Campbell.
"Humans are exceptionally adept at unsupervised learning, and we must make significant strides in this direction to develop AI on par with humans."
— Professor and researcher in deep learning at the University of Montreal, Yoshua Bengio
However, the majority of AI futurists view pure unsupervised learning as the holy grail of deep learning and concede we have a long way to go before we can utilize it to train real AI systems. Deep learning models taught using a technique that falls between supervised and unsupervised learning will likely drive the next generation of AI innovation.
Computer scientists and engineers are investigating a variety of these learning approaches, some of which provide a triple threat: fewer labeled data, a smaller data volume, and less human participation. "one-shot learning" is the method closest to unsupervised learning. It is founded on the concept that the majority of human learning occurs in only one or two instances.
James DiCarlo, chairman of the MIT department of brain and cognitive sciences, cites object recognition as a prime example. "Imagine picking up a cup and rotating it in front of yourself. From the perspective of your visual system, this is unlabelled data. Even without a label for the word 'cup,' it may be utilized to begin teaching the visual system. So it is conceivable that a machine's deep network might be constructed in an entirely uncontrolled manner. On the rear end, there is only a thin layer of labelling. That is the paradigm that many people envision."
Other potential approaches need additional supervision but might assist accelerate and scale deep learning applications anyway. These consist of:
-
• Reinforcement learning: "As the system interacts with an environment, it is rewarded for good behaviour and punished for poor behaviour. This requires supervision, but it is considerably simpler to supply," argues IBM's Campbell.
-
• Transfer learning: "You take a trained model and utilize a small amount of training and labelled data to apply it to a whole different problem," explains IBM's Smith.
-
• Active learning: The algorithm only seeks further labelled data when it requires it. "It's a baby step toward unsupervised learning in the sense that the computer is beginning the labelling as opposed to humans providing the machine tons of labelled data," explains IBM's Soffer.
Effective algorithms and cutting-edge AI hardware
Simplifying the learning process would also alleviate the power constraint that hinders both creativity and the performance of AI applications. GPUs have expedited the training and execution of deep learning models, yet they are insufficient. "It requires a great deal of computer power to train the model and then use it after training. "We may run a test on a model, and it will take two to three weeks to train it. If you're trying to iterate quickly, it's a bit of a problem," says Jason Toy, CEO of Somatic, a firm that develops AI-powered creativity applications. Therefore, we invest a great deal of effort in research and testing to make model designs smaller and quicker.
Bekas of IBM demonstrates why we cannot scale enough hardware to tackle this problem. "In the end, hardware cannot compete with computing complexity. "A mix of algorithmic enhancement and hardware development is required," explains Bekas.
With model enhancements, researchers believe GPUs will gain speed and remain a vital component of the "computational power" variable that drives future AI advances. However, some AI hardware now in development, such as neuromorphic chips or even quantum computing systems, might play into the new formula for AI progress.
In the end, researchers aspire to construct future AI systems that do more than imitate human thought processes such as reasoning and perception; they envision them engaging in an altogether new sort of thinking. This may not occur in the next wave of AI innovation, but AI thought leaders have it in their sights.
"Birds fly by flapping their wings, but for humans to fly, we had to construct a non-natural kind of flight. Similarly, AI will enable us to create numerous new sorts of thinking that do not exist physiologically and are unlike human thought. Therefore, artificial intelligence does not replace human reasoning, but rather enhances it." According to Kevin Kelly, Wired co-founder and bestselling author of The Inevitable.
Written by:
Henry Apaw - CTO, Full stack Designer/Developer @CopyCoach - creating campaigns and software tools for brands and ad agencies for over 13 years.
For all our Blogs Visit Here or Drop me a message at h.apaw@fillmore-xr.com
Further Online Resources
The Step-by-Step Guide to Copywriting
Online Learning and Course Design: Copywriters Toolbox, Volume 1
The Adweek Copywriting Handbook
The Ultimate Guide to Writing Powerful Advertising and Marketing Copy from One of Americas Top Copywriters
Copywriting Made Simple
How to Write Powerful and Persuasive Copy that Sells